Data and responsibility: What is an intersectional approach to data?
To reduce inequalities and practice inclusive development, we must first understand the discrimination or disadvantages people face. Different aspects of a person’s identity – such as their ethnicities, genders, religions, disabilities, or sexual orientations – shape their experiences of advantage/disadvantage, and discrimination/privilege. Unpicking intersecting inequalities involves looking at how aspects of identity overlap to create and deepen discrimination, applying an intersectional lens
Intersectionality is a way of looking at the world – and at data. Data is an essential resource that can enable inclusive development. However, uncritical data practice can have serious consequences for people who are marginalized, further compounding or perpetuating disadvantages and discrimination. Intersectional approaches to data examine the multi-dimensionality of identity and its impacts, fostering positive social transformation.
What is an intersectional approach to data?
An intersectional approach to data identifies inequalities within and between groups of people based on how multiple factors of a person’s identity come together. However, it goes beyond this to ensure that data plays a part in reducing inequality. This includes using intersectionality as a lens to reflectively examine data practice, data processes, and institutions. Intersectional approaches build on the human rights-based data principles of participation, data disaggregation, self-identification, transparency, privacy, and accountability. Intersectional approaches encompass one way to operationalize these important principles in practice.
What does it mean to be marginalized?
The most marginalized and excluded people in data are those who remain completely unknown, silent, muted, unheard, and ignored. Finding and including these people requires different strategies across the data value chain. Intersectionality helps us to see that individuals who are being marginalized are not a fixed group of people or an identity. Marginalization is a process, and refers to the way or the extent that a person experiences disadvantage, discrimination, or social exclusion. However, one of the most important things to recognize is that marginalization is often maintained through structural inequality – such as through institutional racism, classism, or sexism. The UN increasingly recognizes that girls and women, persons living with disabilities, ethnic minorities and indigenous peoples, and refugees and displaced persons are at greater risk of marginalization. Intersectionality research has shown that location, class, employment, and marital status, among other factors, can also influence susceptibility to marginalization.
Author
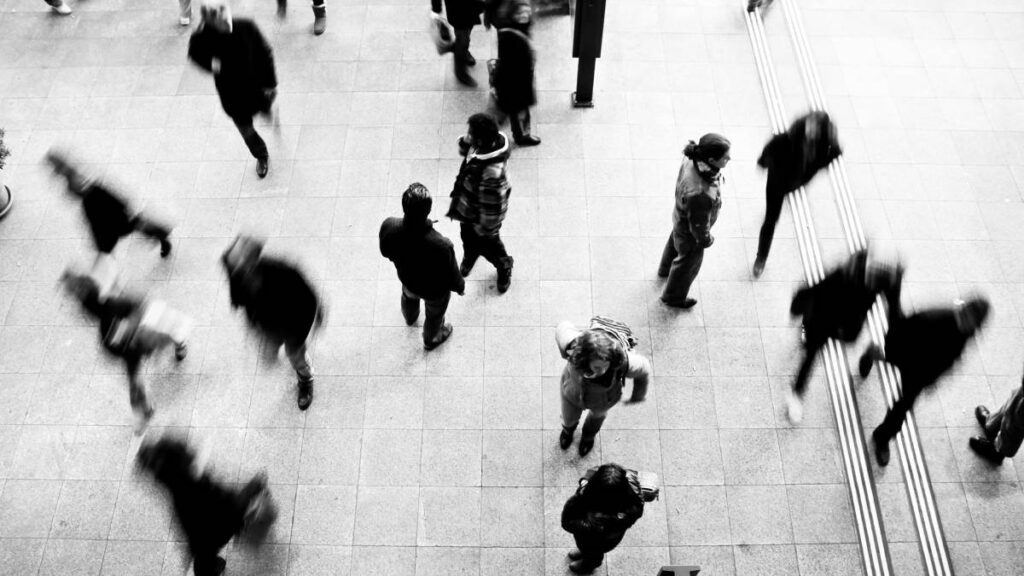
What does it mean to center the voices of individuals at greatest risk of marginalization or discrimination in data?
Centering voices means accepting that lived experience is a valid form of evidence of inequality or discrimination. It means finding those individuals who are most impacted by inequality or discrimination and including them in processes to identify solutions, develop organizational projects/programs, or create policy. It also means including those people in all of the data work that surrounds these activities. Promoting equity across the entire data value chain The data value chain describes the process through which data creates value. For intersectional approaches, data must promote equity for people who face the greatest risk of marginalization or discrimination. The value chain has four major stages: collection, publication, uptake, and impact. These four stages are further separated into twelve steps: identify, collect, process, analyze, release, disseminate, connect, incentivize, influence, use, change, and reuse. By using an intersectional approach, you may uncover the barriers that individuals with certain personal characteristics face. Discovering these will help gain greater consciousness and awareness of intersecting inequality across the data value chain.
Ensuring that institutional data systems are inclusive and safe Intersectional approaches work to make institutional data systems more inclusive. Institutional data systems include all of the tools, processes, and policies that influence how practitioners carry out their work with data. Data systems are designed to take into account the implications of different kinds of data: whether it be textual, numbers, image or sound-based data, digital or analog. Management of data systems also includes management of equipment, safety, security, and access. Data systems show how the various elements of the data value chain come together in an iterative way, as opposed to a linear progression. Making activities within the data value chain inclusive for a specific project or activity may require unique changes. However, making data systems inclusive will require changes that are more standardized or systemic.
Engaging data to increase context awareness and reduce inequality Intersectional approaches to data should be adopted by governments and/or organizations to improve the quality of life of people who have been compromised by intersecting inequality. Such approaches specifically focus on how to meet the needs of people who are marginalized and how entire communities can be supported in that context. Intersectional approaches also emphasize particular impacts, such as the reduction of inequality or discrimination at individual and systemic levels.
Building inclusive institutions One of the main concerns in intersectional approaches to data is that governments and organizations must reflect on how their institution fosters inclusive data practice. To do this, a high priority must be placed on diversity and inclusion in the workforce.
Unconscious bias also runs through institutions, which is one reason why addressing it is crucial. Everyone holds unconscious beliefs about social stereotypes and the groups of people they are commonly applied to. It is essential to critically assess how your own gender, race, class, and other aspects of your identity shape the data you collect, analyze, and use, and apply this to your approach to data that has already been collected. This means strategies to mitigate unconscious bias must take place at an institutional level.
People working in data science, statistics, and other roles in research and practice that use data are highly influential to sustainable development. Individuals with the greatest risk of marginalization or discrimination tend to be represented less in these professions globally, which in turn limits their participation in institutional decisionmaking and impact. Intersectional approaches emphasize increasing diversity and inclusion in the workforce to reduce this inequality.
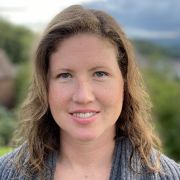
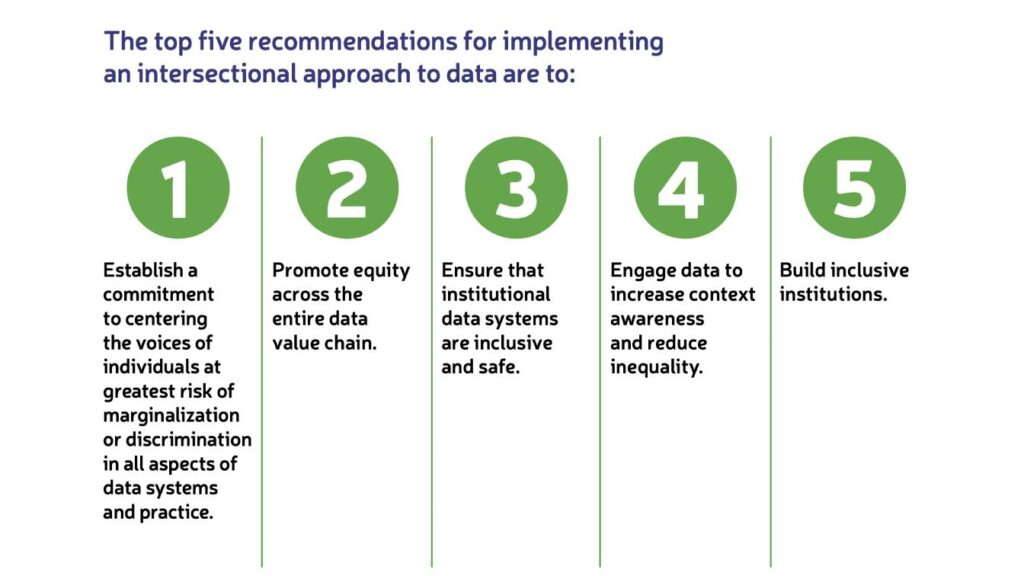
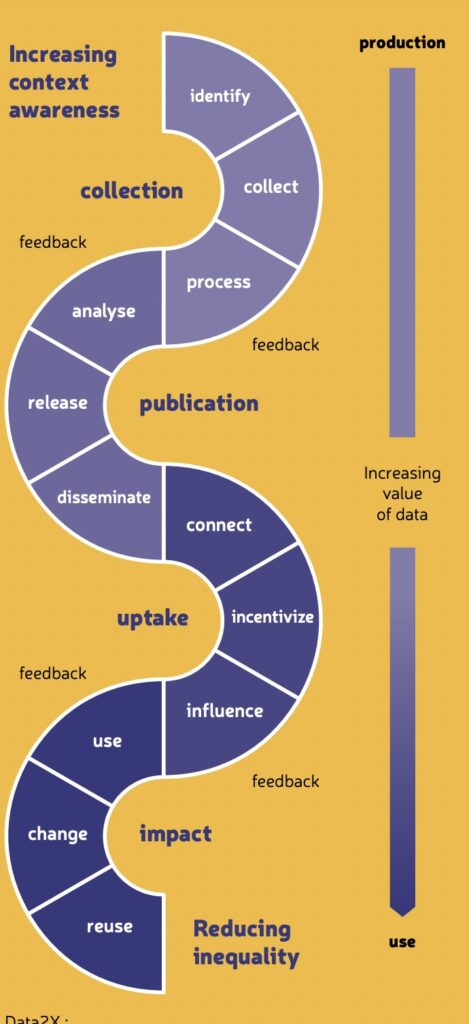
Read more about ‘Unpacking Intersectional Approaches to Data’ | Global Partnership for Sustainable Development Data.